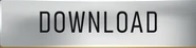
Neighbor classifier based on Dynamic Time Warping. Over the embeddings given by a domain-specific RNN, as well as (ii) a nearest Yields significantly better performance compared to (i) a classifier learned Vehicles, we observe that a classifier learned over the TimeNet embeddings
TIMENET GRU ARCHIVE
For several publicly availableĭatasets from UCR TSC Archive and an industrial telematics sensor data from
TIMENET GRU SERIES
Useful for time series classification (TSC). Representations or embeddings given by a pre-trained TimeNet are found to be Once trained, TimeNet can be usedĪs a generic off-the-shelf feature extractor for time series. Series from several domains simultaneously. To generalize time series representation across domains by ingesting time Rather than relying on data from the problem domain, TimeNet attempts Using sequence to sequence (seq2seq) models to extract features from time Neural network (RNN) trained on diverse time series in an unsupervised manner Generic feature extractors for images, we propose TimeNet: a deep recurrent
TIMENET GRU PDF
If you find this repo useful, please cite our paper.Download a PDF of the paper titled TimeNet: Pre-trained deep recurrent neural network for time series classification, by Pankaj Malhotra and 4 other authors Download PDF Abstract: Inspired by the tremendous success of deep Convolutional Neural Networks as See our paper for the comprehensive benchmark. List of Special Codes CB: Called back must be used to indicate an employee who is on call was called back to work during the on call period. Till February 2023, the top three models for five different tasks are: Model of 13 The clocking entered will be displayed on the Time Card Screen. More than 15 advanced baselines are compared. In this paper, we also provide a comprehensive benchmark to evaluate different backbones. Many Git commands accept both tag and branch names, so creating this branch may cause unexpected behavior.
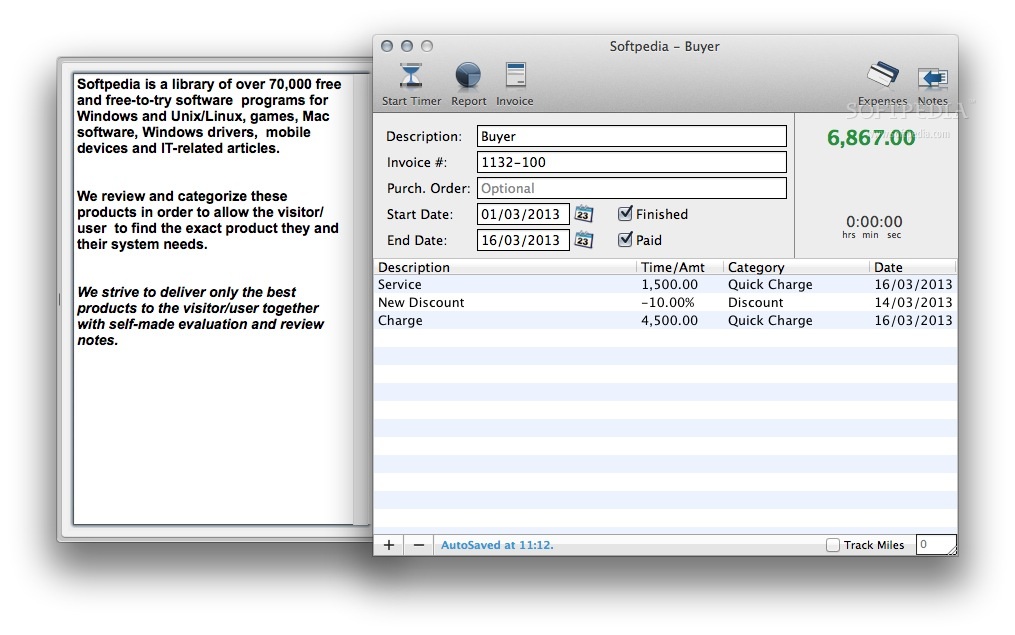
TIMENET GRU CODE
🚩 The complete code and scripts of TimesNet have been included in.
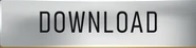